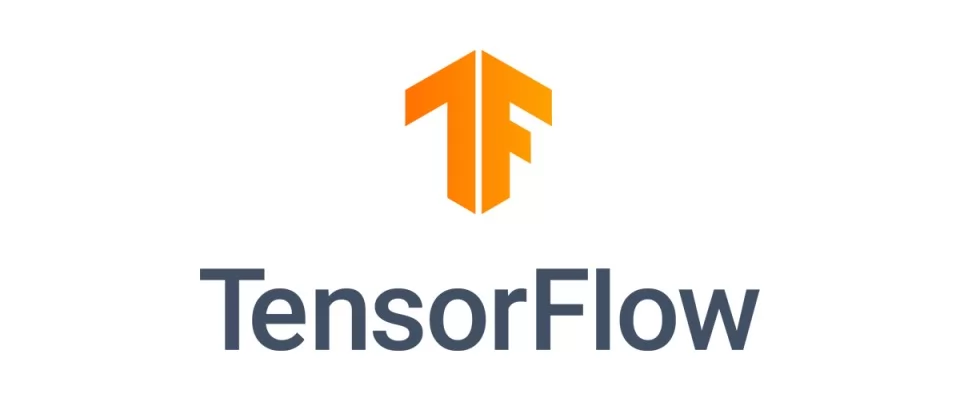
In March the TensorFlow team has released two new packages: facemesh and handpose for tracking key landmarks on faces and hands respectively. This release has been a collaborative effort between the MediaPipe and TensorFlow.js teams within Google Research.
The facemesh package finds facial boundaries and landmarks within an image, and handpose does the same for hands. These packages are small, fast, and run entirely within the browser so data never leaves the user’s device, preserving user privacy. You can try them out right now using these links:
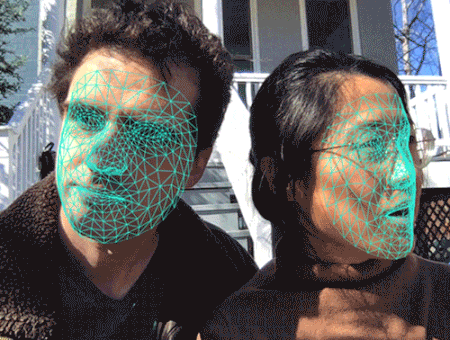
Facemesh package
Handpose package
These packages are also available as part of MediaPipe, a library for building multimodal perception pipelines:
THe TensorFlow.js team hopes real time face and hand tracking will enable new modes of interactivity. For example, facial geometry location is the basis for classifying expressions, and hand tracking is the first step for gesture recognition. They're excited to see how applications with such capabilities will push the boundaries of interactivity and accessibility on the web.Deep dive: Facemesh
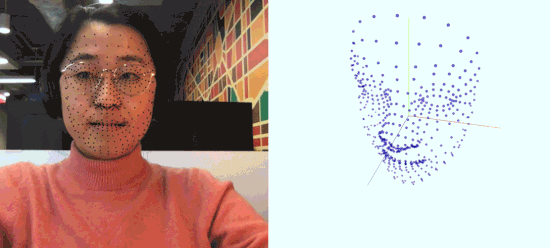
The facemesh package infers approximate 3D facial surface geometry from an image or video stream, requiring only a single camera input without the need for a depth sensor. This geometry locates features such as the eyes, nose, and lips within the face, including details such as lip contours and the facial silhouette. This information can be used for downstream tasks such as expression classification (but not for identification). Refer to TensorFlow.js model card for details on how the model performs across different datasets. This package is also available through MediaPipe.
Performance characteristics
Facemesh is a lightweight package containing only ~3MB of weights, making it ideally suited for real-time inference on a variety of mobile devices. When testing, note that TensorFlow.js also provides several different backends to choose from, including WebGL and WebAssembly (WASM) with XNNPACK for devices with lower-end GPU's. The table below shows how the package performs across a few different devices and TensorFlow.js backends:
Installation
There are two ways to install the facemesh package:- Through NPM:
import * as facemesh from '@tensorflow-models/facemesh;
- Through script tags:
<script src="https://cdn.jsdelivr.net/npm/@tensorflow/tfjs-core"></script> <script src="https://cdn.jsdelivr.net/npm/@tensorflow/tfjs-converter"></script> <script src="https://cdn.jsdelivr.net/npm/@tensorflow-models/facemesh"></script>
Usage
Once the package is installed, you only need to load the model weights and pass in an image to start detecting facial landmarks:// Load the MediaPipe facemesh model assets. const model = await facemesh.load(); // Pass in a video stream to the model to obtain // an array of detected faces from the MediaPipe graph. const video = document.querySelector("video"); const faces = await model.estimateFaces(video); // Each face object contains a `scaledMesh` property, // which is an array of 468 landmarks. faces.forEach(face => console.log(face.scaledMesh));The input to
estimateFaces
can be a video, a static image, or even an ImageData interface for use in node.js pipelines. Facemesh then returns an array of prediction objects for the faces in the input, which include information about each face (e.g. a confidence score, and the locations of 468 landmarks within the face). Here is a sample prediction object:
{ faceInViewConfidence: 1, boundingBox: { topLeft: [232.28, 145.26], // [x, y] bottomRight: [449.75, 308.36], }, mesh: [ [92.07, 119.49, -17.54], // [x, y, z] [91.97, 102.52, -30.54], ... ], scaledMesh: [ [322.32, 297.58, -17.54], [322.18, 263.95, -30.54] ], annotations: { silhouette: [ [326.19, 124.72, -3.82], [351.06, 126.30, -3.00], ... ], ... } }Refer to TensorFlow.js README for more details about the API.
Deep dive: Handpose
The handpose package detects hands in an input image or video stream, and returns twenty-one 3-dimensional landmarks locating features within each hand. Such landmarks include the locations of each finger joint and the palm. In August 2019, TensorFlow.js team released the model through MediaPipe - you can find more information about the model architecture in TensorFlow.js blogpost accompanying the release. Refer to their model card for details on how handpose performs across different datasets. This package is also available through MediaPipe.Performance characteristics
Handpose is a relatively lightweight package consisting of ~12MB weights, making it suitable for real-time inference. The table below shows how the package performs across different devices:
Installation
There are two ways to install the handpose package.- Through NPM:
import * as handtrack from '@tensorflow-models/handpose;
- Through script tags:
<script src="https://cdn.jsdelivr.net/npm/@tensorflow/tfjs-core"></script> <script src="https://cdn.jsdelivr.net/npm/@tensorflow/tfjs-converter"></script> <script src="https://cdn.jsdelivr.net/npm/@tensorflow-models/handpose"></script>
Usage
Once the package is installed, you just need to load the model weights and pass in an image to start tracking hand landmarks:// Load the MediaPipe handpose model assets. const model = await handpose.load(); // Pass in a video stream to the model to obtain // a prediction from the MediaPipe graph. const video = document.querySelector("video"); const hands = await model.estimateHands(video); // Each hand object contains a `landmarks` property, // which is an array of 21 3-D landmarks. hands.forEach(hand => console.log(hand.landmarks));As with
facemesh
, the input to estimateHands can be a video, a static image, or an ImageData interface. The package then returns an array of objects describing hands in the input. Here is a sample prediction object:
{ handInViewConfidence: 1, boundingBox: { topLeft: [162.91, -17.42], // [x, y] bottomRight: [548.56, 368.23], }, landmarks: [ [472.52, 298.59, 0.00], // [x, y, z] [412.80, 315.64, -6.18], ... ], annotations: { indexFinger: [ [412.80, 315.64, -6.18], [350.02, 298.38, -7.14], ... ], ... } }Refer to the TensorFlow.js README for more details about the API.
Looking ahead
The TensorFlow.js team plans to continue improving facemesh and handpose. They will add support for multi-hand tracking in the near future. The team is also always working on speeding up their models, especially on mobile devices. In the past months of development, The TensorFlow team have seen performance for facemesh and handpose improve significantly, and believe this trend will continue. The MediaPipe team is developing more streamlined model architectures, and the TensorFlow.js team is always investigating ways to speed up inference, such as operator fusion. Faster inference will in turn unlock larger, more accurate models for use in real time pipelines.Next steps
- Try out the models
- Learn more about facemesh from this Google Research paper: https://arxiv.org/abs/1907.06724
- Check out this Google AI blogpost announcing the release of facemesh as part of the Android AR SDK: https://ai.googleblog.com/2019/03/real-time-ar-self-expression-with.html
- Learn more about handpose as part of MediaPipe on this Google AI blogpost: https://ai.googleblog.com/2019/08/on-device-real-time-hand-tracking-with.html
Acknowledgements
The TensorFlow team worked together with the MediaPipe team, who generously shared their original implementations of these packages. MediaPipe developed and trained the underlying models, and designed the post-processing graph that brings everything together.
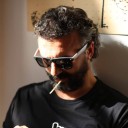
Luigi Nori
He has been working on the Internet since 1994 (practically a mummy), specializing in Web technologies makes his customers happy by juggling large scale and high availability applications, php and js frameworks, web design, data exchange, security, e-commerce, database and server administration, ethical hacking. He happily lives with @salvietta150x40, in his (little) free time he tries to tame a little wild dwarf with a passion for stars.
Related Posts
Deepfakes Detection – An Emerging Technological Challenge
It is highly probable that while browsing the Internet, everyone of us has at some point stumbled upon a deepfake video. Deepfakes usually depict well-known people doing really improbable things…
First steps into JavaScript – a practical guide 3
After we learned the basic ofaccessing DOM elementsandhow to modify them,we are ready for the more exciting parts – handling DOM events. This allows us to make our web way more…
Should We Have This Meeting? - by Wrike project management tools
Infographic brought to you by Wrike project management tracking tool
Face detection methods and classes in php
Face detection is a computer technology that determines the locations and sizes of human faces in arbitrary (digital) images. It detects facial features and ignores anything else, such as buildings,…